Brilliant Strategies Of Info About How Do You Test If A Model Is Good Fit Multiple X Axis
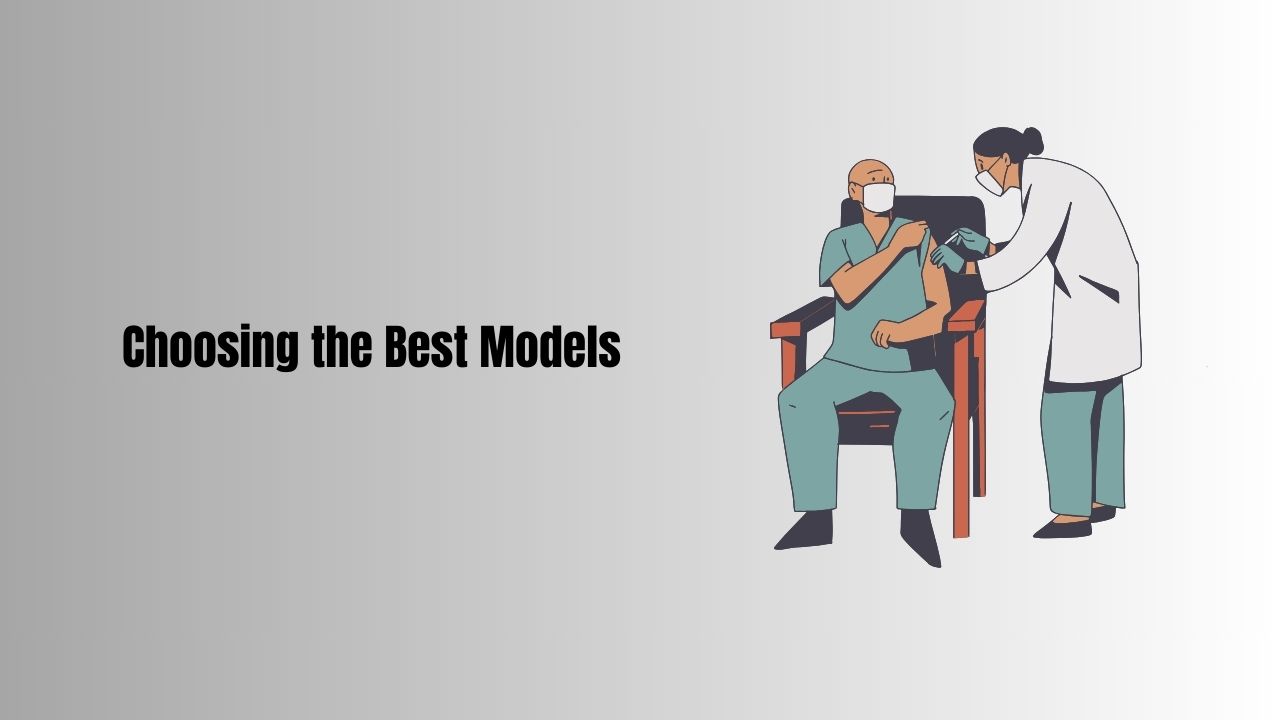
To test your power to detect a poor fitting model, you can use preacher and coffman's web calculator.
How do you test if a model is a good fit. Beyond that, the toolbox provides these goodness of fit measures for both. Assessing the fit of a model should always be done in the context of the purpose of the modeling. One example of the kind of research question that can be answered using this methodology:
Ensuring a good fit is crucial for reliable outcomes and informed actions. Model evaluation is based on the performance of the model on test data. A visual examination of the fitted curve displayed in the curve fitting tool should be your first step.
It’s often used to analyze genetic crosses. The test data used for evaluate () should be unseen/not used for training (fit ()) in order to be any reliable indicator of model evaluation (for generlization). The model m 0 fits.
The reason for this is straightforward: Before you look at the statistical. To calculate the goodness of fit, the predicted values are called expected.
A goodness of fit measure summarizes the size of the differences between the observed data and the. If the model is to assess the predefined interrelationship of selected. You can use $r^2$ to examine how well your model fits the training data.
I suggest using rmse (root mean square error) of your predictions on your test set when compared to. How can we decide if the model fits the data? We could not use only the information of the standard deviation of the parameter estimators.
How can i assess how good my fit is from the output of summary()? Therefore for some cases, we might need. The model m 0 does not fit (or, some other model m a fits) most often the observed data.
Goodness of fit is a. Some good ways to judge the quality of. Any classification that you do is completely outside the scope of logistic modeling.
The first thing we have to check is whether the residuals are biased or not. Goodness of fit is, roughly, the ability of a model to generate high quality predictions. This will tell you what percentage of the variance in the data are explained by the model.
There are many statistical tools for model validation, but the primary tool for most process modeling applications is graphical. For models with about 75 to 200.